BLOG | Call Center Productivity
What is Sentiment Analysis?
Principles and How It Improves
Customer Experience
Each business always aims to satisfy customers’ needs, but not everyone knows about sentiment analysis. In the vast field of the digital world, where information flows ceaselessly and opinions are expressed with a keystroke, understanding the pulse of human sentiment has become more crucial than ever. That is why we cannot underestimate the use of omnichannel analytics to build a complete picture of the market’s perception of your offers.
We embark on an exploration to cover the principles that underlie sentiment analysis, where each interaction with customers plays a key role in the improvement of their experience. Our task is to highlight the core aspects of sentiment analysis with all the pros and cons. Let’s get down to business!
What is Sentiment Analysis?
Sentiment analysis, also referred to as opinion mining, is a computational process within the field of natural language processing (NLP) that aims to discern and evaluate sentiments, opinions, and emotions expressed in written or spoken text.
The primary objective of sentiment analysis is to categorize the subjective content of the language into sentiments such as positive, negative, or neutral. Sentiment analysis seeks to comprehend the nuances of human expression, considering factors like context, tone, and cultural influences by employing advanced algorithms and linguistic analysis.
This analytical approach has widespread applications, ranging from monitoring social media platforms for brand perception to analyzing customer feedback. Remember to analyze calls to improve the customer experience.
Ultimately, all of these factors are insights that businesses can leverage to enhance decision-making processes and overall customer experience.
Principles of Sentiment Analysis
The principles of sentiment analysis revolve around the systematic examination of language to decipher and categorize the sentiments, opinions, and emotions expressed in text data. They extend to real-world applications, such as social media monitoring, customer feedback analysis, and brand reputation management. Also, machine algorithms and natural language processing techniques play a vital role in identifying patterns and making more accurate predictions.
The iterative process of data labeling and training further refines these models, contributing to their accuracy and adaptability across diverse linguistics. As sentiment analysis continues to evolve, its applications become increasingly vital for businesses seeking to navigate the intricate
Text Understanding: In-depth comprehension of language nuances, including context, tone, and subtle expressions, to accurately interpret sentiments.
- Machine Learning and NLP: Integration of machine learning algorithms and natural language processing techniques to automate the analysis of sentiments in textual data.
- Data Labeling and Training: Utilization of labeled datasets to train sentiment analysis models, involving continuous improvement and refinement.
- Contextual Sensitivity: Consideration of the contextual aspects of language, recognizing that the meaning of words and phrases can vary based on the context in which they are used.
- Real-Time Analysis: Capability to analyze sentiments in real-time, allowing for prompt responses and decision-making, particularly in dynamic environments like social media.
- Multilingual Understanding: Adaptation to different languages and cultural expressions, ensuring that sentiment analysis models can accurately analyze sentiments across diverse linguistic landscapes.
- Emotion Recognition: Recognition and classification of a wide range of emotions expressed in text, going beyond basic positive, negative, or neutral categorizations.
- Bias Mitigation: Ongoing efforts to identify and mitigate biases in sentiment analysis models, ensuring fair and accurate results across diverse user groups.
- Continuous Improvement: Embracing a mindset of continuous improvement, with regular updates to models based on new data and emerging linguistic patterns.
- Application Diversity: Application of sentiment analysis across various domains, including social media monitoring, customer feedback analysis, and brand reputation management.
Methods of Sentiment Analysis
To harness the power of sentiment analysis for enhancing customer experience, businesses need to employ effective methods that can accurately capture and interpret customer sentiments.
In this section, we will explore five common methods of sentiment analysis: the rule-based approach, machine learning approach, lexicon-based approach, hybrid approach, and aspect-based sentiment analysis. Each method has its strengths and considerations, and choosing the right approach is crucial to ensure accurate and meaningful analysis:
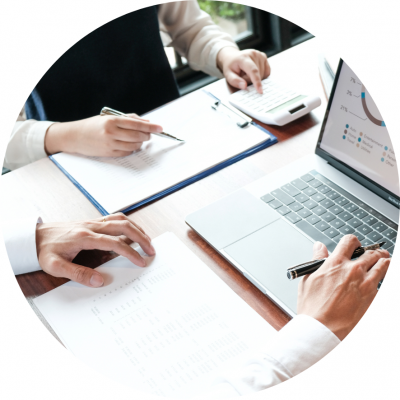
- Rule-based Approach: In this method, sentiment analysis relies on predefined rules and patterns to determine the sentiment of a text. These rules can be based on keywords, linguistic rules, or grammatical patterns. The sentiment of the text is determined by counting the occurrences of positive and negative words or phrases.
- Machine Learning Approach: This approach involves training a machine learning model on a labeled dataset of text samples with their corresponding sentiments. The model learns patterns and relationships in the data and can then predict the sentiment of new, unlabeled text. Popular machine learning algorithms used in sentiment analysis include Naive Bayes, Support Vector Machines (SVM), and Recurrent Neural Networks (RNN).
- Lexicon-based Approach: The method consists of sentiment lexicons or dictionaries that contain words or phrases along with their associated sentiment scores. Each word is assigned a positive, negative, or neutral sentiment score based on its semantic meaning. The sentiment of a text is then calculated by aggregating the sentiment scores of the words or phrases present in the text.
- Hybrid Approach: A combination of multiple methods can be used to improve the accuracy of sentiment analysis. For example, a hybrid approach may use a rule-based method to identify sentiment-bearing words and phrases, and then use a machine learning model to classify the overall sentiment of the text.
- Aspect-based Sentiment Analysis: This method focuses on analyzing the sentiment towards specific aspects or features of a product, service, or brand. It involves identifying and extracting the aspects from the text and then determining the sentiment associated with each aspect separately.
Pros and Cons of Using a Sentiment Analysis System
It is high time to comprehensively explore the intricacies of sentiment analysis, unveiling its potential pros and potential cons. As with any strategic approach, businesses must carefully evaluate both the advantages and disadvantages when incorporating this approach into their operations.
This analytical tool, harnessing the power of language interpretation and machine learning, offers a nuanced understanding of customer sentiments. However, a balanced perspective is crucial as we navigate through the complexities of sentiment analysis, ensuring a thoughtful and informed integration that aligns with the broader objectives of the business.
Pros of Using This System:
- Customer Insight: Gain valuable insights into customer opinions, preferences, and sentiments, increasing understanding of customer needs and expectations.
- Brand Monitoring: Monitor and manage brand perception in real-time, mitigating potential reputation risks, and capitalizing on positive sentiment.
- Data-Driven Decision Making: Facilitate data-driven decision-making processes, enhancing strategic planning based on sentiment trends and analysis.
- Improved Customer Experience: Identify areas for improvement in products and services, boosting overall customer experience by addressing specific issues highlighted through approach.
- Crisis Management: Early detection of negative sentiments allows for proactive crisis management, minimizing the impact of potential crises through timely responses.
- Personalized Marketing: Tailor marketing campaigns based on customer sentiments, amplifying the effectiveness of marketing efforts by aligning with audience preferences.
- Efficient Customer Support: Integrate sentiment analysis into customer support for real-time issue resolution, and improve customer satisfaction through prompt and effective support.
- Competitive Advantage: Stay ahead of competitors by understanding and responding to market sentiments. Leverage sentiment insights for strategic positioning and differentiation.
Cons of Using This System:
- Bias and Accuracy Issues: Automated models may carry biases, leading to inaccurate sentiment predictions, risk misinterpretation, and potential reinforcement of existing biases.
- Complex Language Nuances: Difficulty in accurately interpreting complex language nuances such as sarcasm, irony, or culturally specific expressions.
- Contextual Challenges: Capturing context correctly can be challenging, leading to the potential misinterpretation of sentiments without a comprehensive understanding of the context.
- Multilingual Challenges: Automated models may struggle with sentiment analysis in multilingual contexts, ensuring accuracy across diverse linguistics.
- Over Reliance on Text: Limited ability in non-textual content like images or videosб which gives incompleted insights in multimedia-driven digital content.
- Dynamic Sentiment Trends: Adapting the system to evolving sentiment patterns in real-time due to rapid changes in trends.
- Privacy Concerns: Handling personal data raises privacy considerations. So, adherence to privacy regulations and ethical practices is essential.
- Resource Intensive: Building and maintaining effective sentiment analysis systems can be resource-intensive. Therefore, it requires ongoing investment in technology, training, and data management.
How Does Sentiment Analysis Improve Customer Experience?
Sentiment analysis, powered by artificial intelligence and natural language processing, plays a crucial role in improving customer experience. By analyzing customer feedback, reviews, and social media comments, sentiment analysis provides valuable insights into customer sentiments and emotions. This helps businesses understand how customers feel about their products, services, and brands, enabling them to make data-driven decisions to enhance the overall customer experience.
By identifying negative sentiments in real time, companies can promptly address customer concerns, resolve issues, and provide personalized support. This proactive approach leads to increased customer satisfaction, loyalty, and ultimately, business growth.
Sentiment analysis empowers businesses to better understand their customers and deliver exceptional experiences that meet and exceed expectations.
- Real-Time Feedback Processing: Allows businesses to process customer feedback in real-time, leading to swift identification and resolution of issues, and increased customer satisfaction.
- Proactive Customer Support: Enables proactive responses that anticipate and address customer needs before they escalate, to foster positive interactions.
- Personalized Interactions: Understanding customer sentiments enables personalized communication, increasing interaction and encouraging a sense of individualized attention.
- Product and Service Enhancements: Analyzing feedback guides product and service improvements, aligning offerings with customer expectations. So, it leads to updating the overall experience.
- Tailored Marketing Campaigns: Informing marketing strategies based on customer preferences. As a result, campaigns resonate more effectively, resulting in increased engagement and positive brand perception.
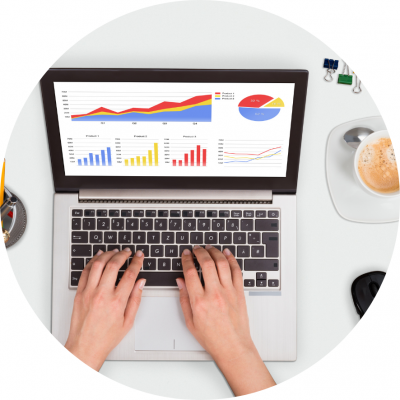
- Brand Reputation Management: Monitoring sentiment helps manage and maintain brand reputation. It means timely responses to negative sentiment mitigate potential damage, while positive sentiment is capitalized for brand promotion.
- Customer Journey Optimization: Identifying pain points and positive touch points allows optimization, creating a seamless and satisfying customer experience.
- Strategic Decision-Making: Data-driven insights from sentiment analysis inform strategic decisions. In consequence, businesses can align their strategies with customer sentiments, ensuring initiatives resonate positively with the target audience.
- Competitive Edge: Utilizing the analysis provides a competitive advantage. Therefore, businesses stay ahead in the market by understanding and responding to customer sentiments more effectively than competitors.
- Continuous Improvement: Regular feedback loops and adjustments based on sentiments contribute to an ever-evolving, customer-focused business model.
Nectar Desk Tools for Better Analyzing of Сustomer Sentiment
Nectar Desk offers a range of tools and features that can contribute to effective sentiment analysis. With its advanced call monitoring and recording features, businesses can analyze customer calls to identify areas of improvement and gain insights into customer sentiment.
The software also facilitates two-way text messaging, allowing businesses to interact with customers via text messages and gather valuable feedback. Additionally, Nectar Desk’s real-time analytics and performance metrics provide businesses with the necessary data to measure customer satisfaction and sentiment.
These tools help in understanding customer emotions and preferences, enabling businesses to enhance the overall customer experience:

- Automatic Call Distribution (ACD): Nectar Desk’s ACD system efficiently routes incoming calls to the right agents, ensuring faster response times and improved customer satisfaction;
- Interactive Voice Response (IVR): Our IVR system streamlines call handling by allowing customers to navigate menus and access information without agent intervention;
- Call Recording: Nectar Desk provides call recording capabilities, allowing you to monitor and review customer interactions for quality control and compliance;
- Real-Time Analytics: Access to real-time data and analytics empowers you to make informed decisions, optimize call center performance, and identify areas for improvement;
- Outbound Calling: Nectar Desk’s outbound calling feature helps your team proactively engage with customers, nurturing relationships and driving sales opportunities;
Wrapping up
To sum up, sentiment analysis plays a crucial role in improving the customer experience. By analyzing customer sentiment, businesses can gain valuable insights into customer behavior, preferences, and emotions. This understanding allows them to tailor their strategies, products, and services to meet customer expectations.
With the help of sentiment analysis tools like Nectar Desk, businesses can identify areas of improvement, track sentiment trends, and make data-driven decisions. By actively listening to customer feedback and addressing their concerns, businesses can enhance customer satisfaction, build stronger relationships, and ultimately create a positive and memorable customer experience. Sentiment analysis is no longer a luxury but a necessity for businesses looking to stay competitive in today’s customer-centric market.
Have you already tried out Nectar Desk tools to make the most of modern technology and optimize your business?
